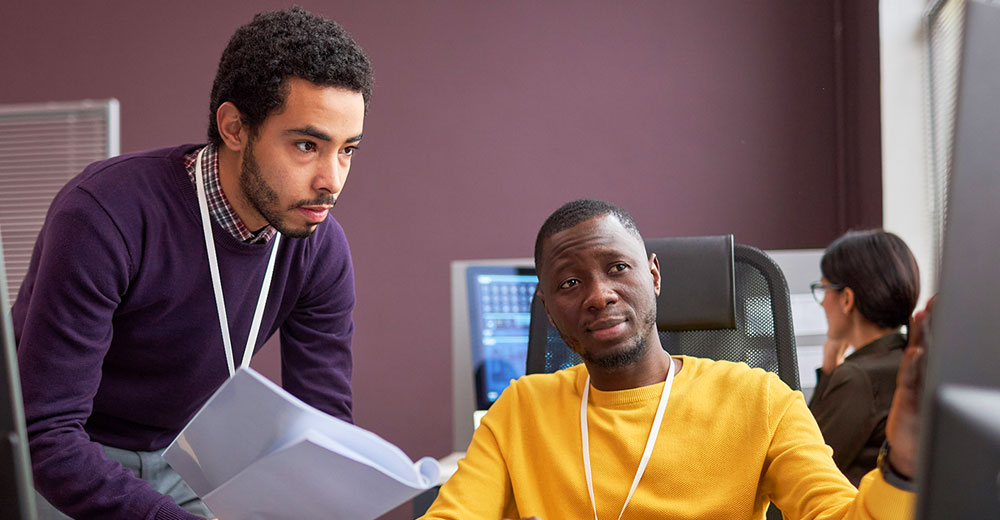
Data poisoning is a cyberattack where malicious or misleading data is inserted into AI training datasets to corrupt their behavior, leading to biased, skewed, or harmful outcomes. A critical threat associated with this attack is the creation of backdoors, which allow for the malicious exploitation of AI and machine learning (ML) systems.
As AI systems become increasingly integrated into essential infrastructure and daily life, this type of cyberattack poses a serious concern for developers and organizations that deploy artificial intelligence technologies.
The field of AI security is advancing quickly, with new threats emerging alongside innovative defense strategies aimed at tackling data poisoning and its countermeasures. A recent report from the managed intelligence company Nisos highlights how bad actors employ various forms of data poisoning attacks, such as mislabeling, data injection, and more complex techniques like split-view poisoning and backdoor manipulation.
The Nisos report points to growing sophistication in these attacks, with adversaries developing targeted and undetectable methods. The report stresses the need for a comprehensive approach to AI security that incorporates technical solutions, organizational policies, and regulatory strategies.
Patrick Laughlin, Senior Intelligence Analyst at Nisos, noted that even a small amount of poisoning—affecting just 0.001% of the training data—can significantly alter AI models’ behavior. Data poisoning attacks can have serious consequences across sectors like healthcare, finance, and national security.
“It highlights the need for a robust mix of technical solutions, organizational policies, and ongoing vigilance to effectively mitigate these risks,” Laughlin told TechNewsWorld.
Current AI Security Measures Falling Short
Laughlin also pointed out that current cybersecurity practices are inadequate in addressing these evolving threats, suggesting the need for new strategies to combat data poisoning.
“It calls for AI-assisted threat detection systems, the development of more resilient learning algorithms, and the integration of advanced technologies such as blockchain to ensure data integrity,” Laughlin added.
The report underscores the importance of privacy-preserving machine learning techniques and adaptive defense systems capable of learning and responding to new attack methods. It also emphasizes that these challenges extend beyond businesses and infrastructure.
Data poisoning attacks can have far-reaching impacts, threatening critical areas such as healthcare, autonomous vehicles, financial markets, and national security. They also have the potential to erode public trust in AI technologies and amplify societal issues, including the spread of misinformation and biases.
Data Poisoning Poses Serious Threats to Critical Systems
Patrick Laughlin warns that one of the most dangerous consequences of data poisoning is its potential to undermine decision-making in critical systems. For example, compromised AI systems in healthcare diagnostics or autonomous vehicles could directly endanger human lives.
The financial sector also faces significant risks, with the possibility of substantial financial losses and market instability due to compromised AI systems. Additionally, the report highlights concerns that a decline in trust towards AI systems could hinder the adoption of beneficial technologies.
“National security risks could arise, exposing critical infrastructure to vulnerabilities and enabling large-scale disinformation campaigns,” Laughlin added.
Real-World Examples of Data Poisoning Attacks
The report details several examples of data poisoning attacks. A notable case is the 2016 breach of Google’s Gmail spam filter, which allowed malicious emails to bypass security measures. Another example is the 2016 compromise of Microsoft’s Tay chatbot, which began producing offensive responses after being exposed to malicious training data.
Further examples include vulnerabilities in autonomous vehicle systems, facial recognition technologies, and potential weaknesses in medical imaging classifiers and financial market prediction models.
Strategies to Combat Data Poisoning Attacks
To mitigate the risks of data poisoning, the Nisos report outlines several strategies. Key recommendations include implementing strong data validation and sanitization techniques, continuous monitoring and auditing of AI systems, and employing adversarial sample training to enhance model resilience.
“It also advocates for diversifying data sources, implementing secure data handling protocols, and promoting user awareness and education,” Laughlin stated.
He further recommended that AI developers control and isolate the sourcing of datasets while investing in programmatic defenses and AI-assisted threat detection systems.
Anticipating Future Challenges in AI Security
The report also raises concerns about emerging trends in data poisoning. As cybercriminals become increasingly skilled, more sophisticated and adaptive poisoning techniques are expected to evade current detection methods. The report also highlights potential risks in newer AI approaches, such as transfer learning and federated learning systems, which may introduce additional vulnerabilities.
“These methods could create new attack surfaces,” Laughlin cautioned.
The growing complexity of AI systems also presents challenges in balancing security with other important factors such as privacy and fairness. As the AI landscape evolves, the industry must push for standardized regulations to ensure comprehensive AI security.